机器学习诗词创作_通过机器学习创作音乐
机器学习诗词创作
In Sweden, there’s something called the Allemansrätten, which literally translates to “Everyman’s Right.”
在瑞典,有一个叫Allemansrätten的东西,字面意思是“每个人的权利”。
Allemansrätten gives everyone the right to access, trespass, and camp on any land within the immediate vicinity of a residential dwelling.
Allemansrätten赋予所有人在住宅附近的任何土地上进入,侵入和扎营的权利。
Imagine a stranger strolling onto your property in the U.S. or U.K., pitching a tent on your lawn, and proclaiming “Allemansrätten!”, as they roast marshmallows over an open fire.
想象一个陌生人漫步到您在美国或英国的财产,在您的草坪上搭起帐篷,并宣称“Allemansrätten!” 当他们在明火上烤棉花糖时。
In Sweden, this is legal and they’re allowed to stay for up to 24 hours.
在瑞典,这是合法的,允许他们最多停留24小时。
Allemansrätten is a very Nordic notion of the social contract and it embodies the idea of Trust that is at the root of Swedish culture.
Allemansrätten是一个非常北欧的社会契约概念,它体现了信任的概念,而信任是瑞典文化的根源。
The idea of “public” vs. “private” just doesn’t exist to some extent in Sweden, and thus it is not surprising that Sweden has been a leader in both Music Piracy and Music Innovation.
在瑞典根本就不存在“公共”与“私人”的概念,因此瑞典在音乐盗版和音乐创新领域都处于领先地位也就不足为奇了。
艺术家的时代 (The Age of the Artist)
Music Piracy, with the launch of Napster, defined much of the 2000s and was catalyzed with the adoption of the MP3 technology and the launch of Apple’s iPod in 2001.
随着Napster的发布,《 音乐盗版》定义了2000年代的大部分时间,并受到MP3技术的采用和2001年苹果iPod的推出的推动。
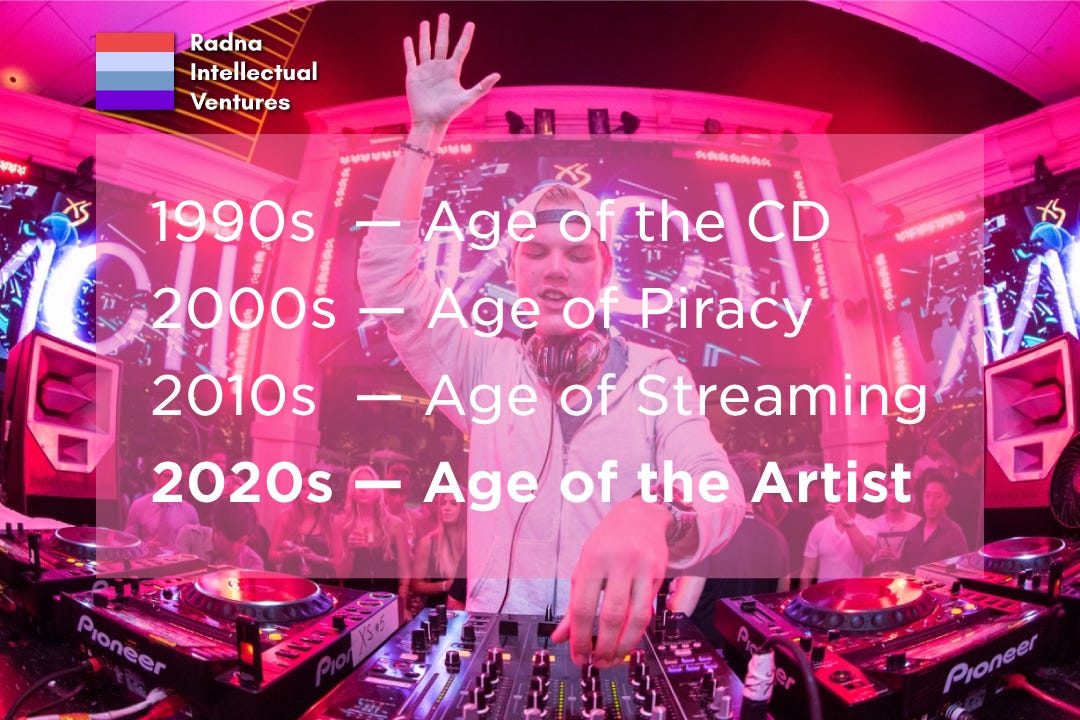
Even with Napster’s shut down by the Recording Industry Association of America (RIAA) in July 2001, the flood gates were opened and nothing could stop Music Piracy, which proliferated in various forms including Sweden’s own Pirate Bay and uTorrent.
即使Napster在2001年7月被美国唱片工业协会 ( RIAA )关闭,防洪闸门也被打开,没有任何东西可以阻止Music Piracy , Music Piracy以各种形式扩散,包括瑞典自己的Pirate Bay和uTorrent 。
A startling statistic: CD Album Sales topped USD 13.4 Billion in 2000 but by 2014, eroded to just 1.9 Billion, a staggering collapse of 86 percent.
令人震惊的统计数据: CD专辑的销售额在2000年突破了134亿美元,但到2014年,仅侵蚀了19亿美元, 跌幅高达86% 。
The 2010s brought with it the exponential growth of Music Streaming through services like Apple iTunes, Amazon Music, and Spotify.
通过苹果iTunes , 亚马逊音乐和Spotify等服务, 音乐流在2010年代呈指数增长。
However, amidst the sea of change in the Music Distribution industry, the underlying relationship between Artists and Labels remains relatively unchanged.
然而,在一片音乐分销业变革的海, 艺术家和唱片公司之间的基本关系保持相对不变。
This brings us to the “Age of the Artist”.
这使我们进入了“艺术家时代” 。
The Age of the Artist represents a structural change in the music industry at the “Top of the Funnel” and will fundamentally shift the relationship between Labels and Artists.
艺术家的时代代表着“漏斗之巅”音乐产业的结构性变化 ,并将从根本上改变唱片公司与艺术家之间的关系。
Alternative Revenue Models: creative deal structures more aligned with Artists than the traditional model of signing artist rights away to a label into perpetuity.
替代收入模型 :与传统的将艺术家权利永久授予标签的签名模型相比,创造性的交易结构与艺术家更一致。
Global Access to Audience: according to legendary VC investor Mary Meeker, 60% of the world’s population is connected to the Internet, and with it access to YouTube, SoundCloud, and a plethora of distribution platforms, allowing any independent artist to share their content and connect with a global audience.
观众的全球访问权 :传奇的VC投资者Mary Meeker表示 ,全球60%的人口连接到Internet,并可以访问YouTube , SoundCloud和众多发行平台,从而允许任何独立的艺术家共享自己的内容和与全球观众建立联系。
Labels as a Service (“LaaS”): similar to the idea of SaaS (“software as a service”) — the subscription-based business model that has disrupted the software industry — I believe LaaS will gain momentum, with Labels becoming more commoditized.
标签即服务(“ LaaS”) :与SaaS (“软件即服务”)的思想类似-一种基于订阅的商业模式,已经破坏了软件行业-我相信LaaS 标签将变得越来越商品化,因此势头将会增强。
With the rapid expansion of creation, collaboration, production, marketing, and distribution tools, the effect is a shift of bargaining power from Labels to Artists.
随着创作 , 协作 , 生产 , 市场营销和发行工具的Swift扩展,其效果是议价能力从“标签”转移到“艺术家” 。
So the Age of the Artist will be defined by a paradigm shift in companies establishing relationships with artists and songwriters during the creation process, setting up the stage for the new label of the future.
因此,艺术家的年龄将由公司在创作过程中与艺术家和词曲作者建立关系的范式转变来定义,从而为未来的新品牌搭建舞台。
Spotify — one of the key disrupters in the $22 Billion Recorded Music industry — agrees with this thesis which we will highlight through their Corporate Development strategy below.
Spotify是220亿美元唱片音乐产业的主要颠覆者之一,我们对此观点表示赞同,我们将在下面的“ 企业发展战略”中对此进行重点介绍。
But before we do, let’s do a quick primer on Product Development and specifically the development of products whose core tech is based on Machine Learning.
但是在开始之前,让我们快速入门产品开发 ,特别是其核心技术基于机器学习的 产品开发 。
机器学习时代的产品开发 (Product Development in the Age of Machine Learning)
One of my first roles after graduating from Cornell University and U.C. Berkeley with degrees in Computer Science was in Product Management at a financial services firm.
从康奈尔大学和加州大学伯克利分校获得计算机科学学位后,我的第一个职位是在一家金融服务公司的产品管理部门。
Back in the early 2010s, “Data Science” and “AI / ML” were still relatively new words in the financial industry, yet our group leverage “Big Data” and utilized Machine Learning algorithms to estimate movements in certain financial markets with remarkable precision.
早在2010年代初期, “数据科学”和“ AI / ML”在金融行业仍是相对较新的词汇,但我们的团队利用“大数据”并利用机器学习算法以极高的精度估算某些金融市场的动向。
The idea of an “AI Product Manager” simply didn’t exist back then, yet that was what our group was doing on a daily basis, which allowed us to generate record profits among our competitors in the industry.
当时根本不存在“ AI产品经理”的想法,但这是我们团队每天都在做的事情,这使我们能够在行业竞争对手中创造创纪录的利润。
Fast forward a decade and the idea of AI Product Management or APM is a clearly defined role in Tech, with the largest tech companies like Amazon, Facebook, and Google even having dedicated APM teams.
十年过去了, AI产品管理或APM的概念在技术领域已明确定义, 亚马逊 , Facebook和Google等最大的科技公司甚至拥有专门的APM团队。

What is the role of an AI Product Manager?
AI产品经理 的角色是什么 ?
The AI Product Manager is principally responsible for using AI, Deep Learning, and Machine Learning to enhance, improve, create, and shape products.
AI产品经理主要负责使用AI,深度学习和机器学习来增强,改进,创建和塑造产品。
Artificial Intelligence has far-reaching implications for every conceivable industry according to a seminal report by McKinsey and Company, which identified over 400 business use cases of AI for companies today.
麦肯锡公司 ( McKinsey and Company)的一份开创性报告显示 , 人工智能对每个可能的行业都有深远的影响,该报告为当今的公司确定了400多个 AI 业务用例 。
Another recent survey of global business leaders and corporate CEOs indicated that over 70% have started AI initiatives. With the proliferation of AI into business (e.g., Google Search, Amazon Product Recommendations, Tesla Autopilot), Machine Learning is the common thread among all these novel products.
最近对全球商业领袖和企业CEO进行的另一项调查表明,超过70%的企业已启动AI计划 。 随着AI进入商业领域(例如Google搜索 , 亚马逊产品推荐 , Tesla Autopilot )的普及,机器学习已成为所有这些新颖产品中的共同点。
Andrew Ng — a Stanford Professor and one of the leading authorities in Machine Learning — developed the concept of the “Virtuous Cycle of AI” — an idea central to the development of Machine Learning products.
斯坦福大学教授,机器学习的主要权威之一安德鲁·伍 ( Andrew Ng)提出了“人工智能的良性循环”的概念 —机器学习产品开发的中心思想。

Andrew’s insight that “building a better AI product requires more users producing more data” has profound implications for the Product Development Life Cycle.
安德鲁的见解“构建更好的AI产品需要更多的用户产生更多的数据”对于产品开发生命周期具有深远的意义。
Why does this matter?
为什么这么重要?
Well, products fueled by data and machine learning can be remarkably powerful tools to discover and solve customer problems, enabling a company to strengthen its business moat amidst an increasingly competitive environment with lower barriers of entry.
好吧,由数据和机器学习推动的产品可以是发现和解决客户问题的非常强大的工具,从而使公司能够在竞争日益激烈且进入壁垒较低的环境中,加强其业务护城河 。
As the diagram below shows, Product Development can be broken into four components, which you can read more about in this Harvard Business Review article.
如下图所示, 产品开发可以分为四个部分,您可以在此《 哈佛商业评论》 文章中了解更多信息。

From the perspective of AI / ML products, here is what these four components really mean.
从AI / ML产品的角度来看,这四个部分的真正含义是。
User Experience: this is where user problems are discovered and validated. As Marc Andreessen of the Venture Capital firm A16Z, notes in “It’s Time to Build”, innovation is sorely missing in Tech. UX / UI — beyond providing an attractive and intuitive user interface — is about discovering what the user really wants and validating the problem, which often leads to product innovation.
用户体验 :这是发现和验证用户问题的地方。 正如风险投资公司A16Z的 马克·安德森 ( Marc Andreessen)在“建立的时机”中指出的那样,创新在技术领域非常缺乏。 UX / UI (除了提供有吸引力的直观用户界面之外)还在于发现用户的真正需求并验证问题,这通常会导致产品创新 。
Technical Development: this covers both software or hardware development. Especially relevant for software is the Agile Software Development methodology, which is particularly well-suited for AI / ML product development.
技术开发 :涵盖软件或硬件开发。 与软件特别相关的是敏捷软件开发方法,该方法特别适合于AI / ML产品开发 。
Data Science: although Data Science is a term that is often associated with buzzwords like “Cloud Computing” and “Big Data”, it has been a concrete field used by the best innovators for decades. Leveraging Machine Learning Algorithms and Big Data, one can truly achieve the extraordinary — see Jim Simmons of Renaissance Technologies — the most successful investor in history (in fact, twice as good as the legendary Warren Buffet as measured by returns).
数据科学 :尽管数据科学是一个经常与诸如“云计算”和“大数据”之类的流行词相关的术语,但数十年来,它一直是最佳创新者使用的具体领域。 利用机器学习算法和大数据,可以真正实现非凡的成就-参见Renaissance Technologies的 Jim Simmons-历史上最成功的投资者(实际上,按回报率衡量,它是传奇的Warren Buffet的两倍)。
Product Management: a Product Manager is akin to the Conductor of an Orchestra. Product Management is an iterative process of gathering user data to build better products, which generates more novel data (see Virtuous Cycle above). An exceptional Product Manager, especially in AI / ML, is someone with a balance of technical expertise + business acumen + high customer empathy.
产品管理 :产品经理类似于管弦乐队的指挥。 产品管理是一个收集用户数据以构建更好的产品的迭代过程,该过程会生成更多新颖的数据(请参见上面的“ 良性循环” )。 一位出色的产品经理,尤其是在AI / ML中,是一位在技术专长+业务敏锐度+高客户同理心之间取得平衡的人。
Given the above, another valuable insight from Andrew Ng is that “Training Sets are the new Wireframes in product management” — a deep observation which you can read more about in Why AI is the New Electricity.
鉴于上述内容,Andrew Ng的另一个有价值的见解是“ 培训集是产品管理中的新线框” –您可以在《 为什么AI是新的电力》中内容。
With an understanding of Product Development in the Age of Machine Learning, let’s exercise our knowledge through a Case Study for one of the most remarkable Machine Learning companies that exist today: Spotify.
了解了机器学习时代的产品开发之后 ,让我们通过案例研究来锻炼我们的知识,该案例研究针对的是当今存在的最杰出的机器学习公司之一: Spotify 。
案例研究:Spotify (Case Study: Spotify)
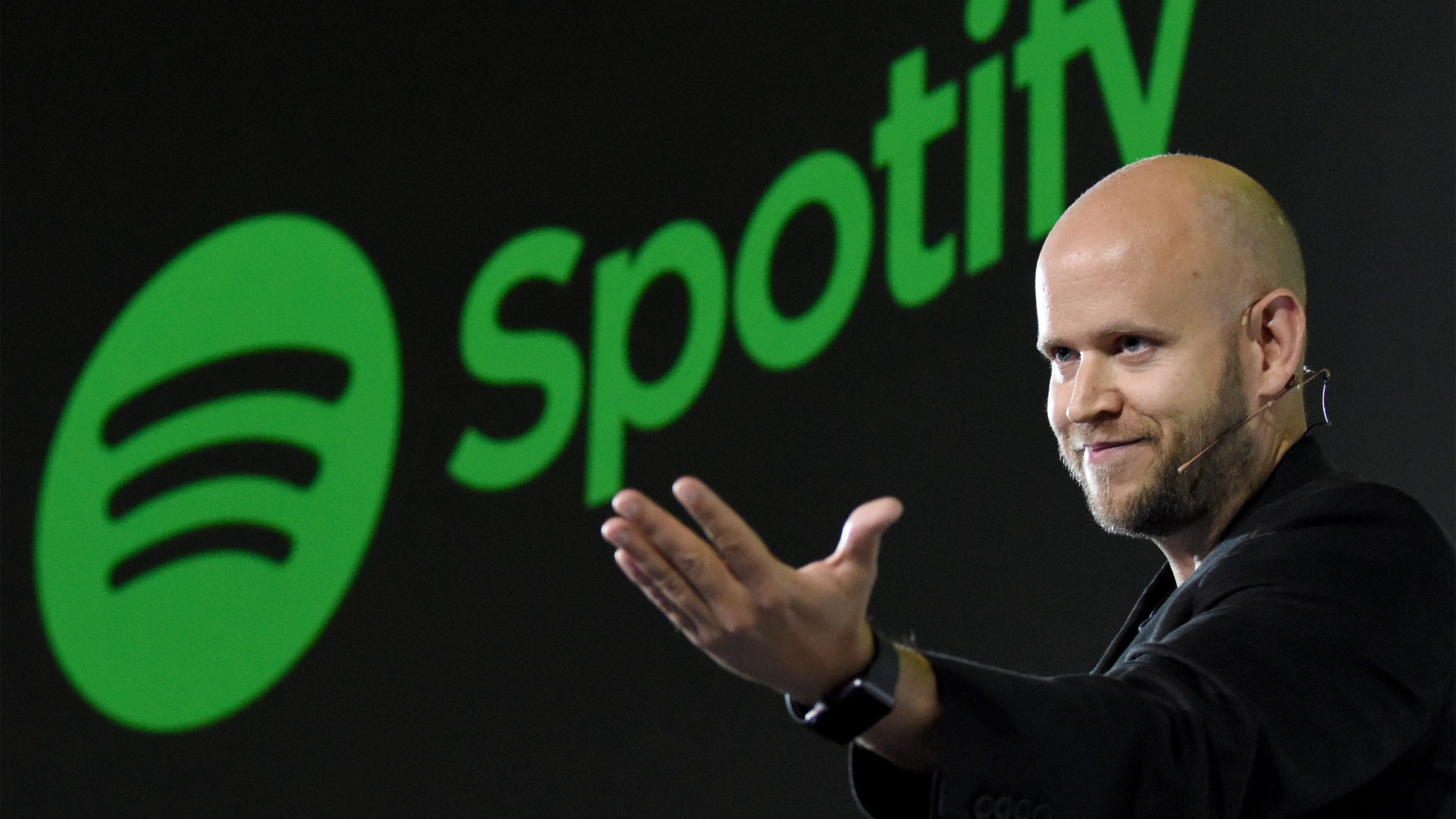
I’d argue Spotify’s history is much more remarkable than Facebook’s story.
我认为Spotify的历史比Facebook的故事更出色。
However, largely as a function of its Swedish origin, Spotify has been less glamorized than Facebook, as epitomized in the popular American film “The Social Network”.
但是,很大程度上归功于其瑞典血统,Spotify的吸引力不及Facebook ,如美国受欢迎的电影“ The Social Network”中的缩影。
What were the humble beginnings of Spotify?
Spotify的卑微起点是什么?
It began in the mind of a music-loving young teenager and prodigy, Daniel Ek.
它始于一个热爱音乐的年轻少年和神童Daniel Ek的想法。
By the age of nine, Daniel taught himself how to code C++ — one of the more challenging programming languages to master — and wrote 40 pages of code, constructing a cursor to move around the screen.
到九岁时,Daniel学会了如何编写C ++ ( 一种最难掌握的编程语言),并编写了40页代码,构造了一个在屏幕上移动的光标。
Daniel continued to hone his coding skills and by age 14 in 1997, Daniel started building websites for other Swedish entrepreneurs, charging a rate of SEK 10,000 per website.
Daniel继续磨练自己的编码技能,到1997年14岁时,Daniel开始为其他瑞典企业家建立网站,每个网站的收费为10,000瑞典克朗。
With this first successful business venture, Daniel caught the entrepreneurial bug, planting the seed for the conception and launch of Spotify nine years later in 2006.
丹尼尔(Daniel)凭借这家首家成功的商业企业,抓住了企业家的臭虫,为概念的创立打下了基础,并在9年后的2006年推出了Spotify。
If you don’t know or use Spotify, I’d urge you to stop here.
如果您不认识或使用Spotify ,请您在此停止。
Download the app on your phone and use it for a week.
在手机上下载该应用程序并使用一周。
As a Zen practitioner, I jokingly compare the Spotify music experience to the journey of discovering Zen, as they are both experiential in nature — we can talk about both, but you must experience them for yourselves in order to truly understand.
作为一名禅宗修炼者,我开玩笑地将Spotify音乐体验与发现禅宗的旅程进行了比较,因为它们都是体验式的 本质上-我们可以讨论这两者,但是您必须亲自体验它们才能真正理解。
Okay, let’s move on to the secret sauce behind Spotify, which relies on Machine Learning.
好的,让我们继续看Spotify背后的秘密之处,后者依赖于Machine Learning 。
Spotify的机器学习 (Machine Learning at Spotify)
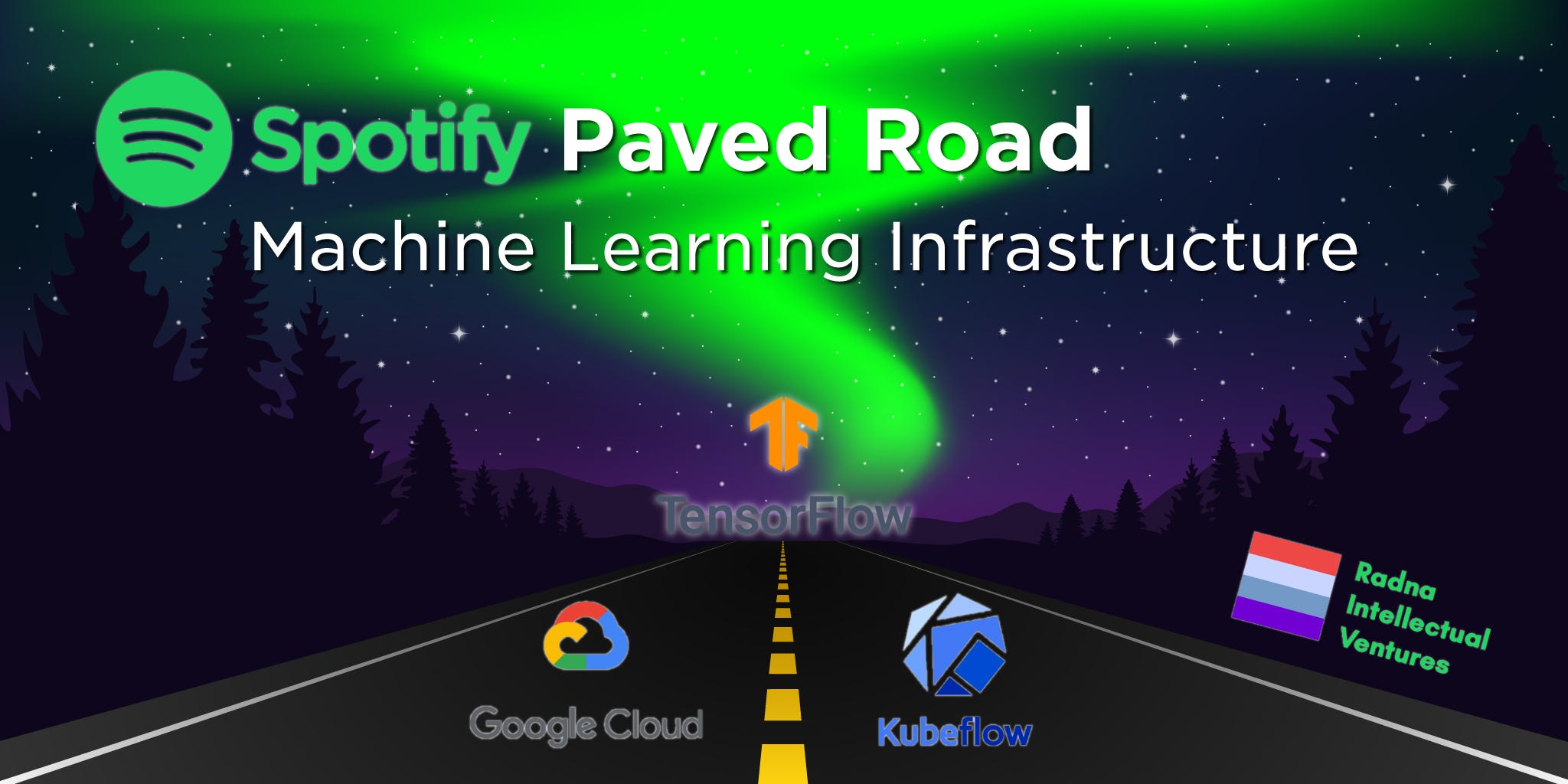
Although Spotify has over 50 million songs, it has a staggering 3 Billion Playlists or 60 times more playlists than songs.
尽管Spotify拥有超过5000万首歌曲 ,但其拥有惊人的30亿个播放列表,或播放列表的数量是歌曲的60倍。
How do we wrap our heads around this?
我们该如何解决呢?
From a Machine Learning perspective, the answer should be clear if you have read the prequel to this article: “The VC’s Guide to Machine Learning”.
从机器学习的角度来看,如果您已阅读本文的前传 : “ VC的机器学习指南” ,答案应该很清楚。
If you haven’t, don’t panic.
如果没有,不要惊慌。
Spotify considers each Playlist as a state space consisting of individual states representing a single song. By leveraging reinforcement learning techniques and user data, Spotify is able to generate novel “Journeys” / Playlists through the vast 50-million dimensional vector space of songs.
Spotify将每个播放列表视为一个状态空间,其中包含代表一首歌曲的各个状态。 通过利用强化学习技术和用户数据,Spotify可以通过浩大的5,000万维向量空间生成新颖的“旅程” /播放列表。
What does that mean in plain English?
普通英语是什么意思?
Simply stated, Spotify’s content recommendation and playlist generation rely heavily on state-of-the-art machine learning algorithms to generate remarkably impactful music recommendations for its 286 million Monthly Active Users (“MAUs”).
简而言之,Spotify的内容推荐和播放列表的生成很大程度上依赖于最新的机器学习算法,以为其2.86亿月活跃用户(“ MAU”)产生非常有影响力的音乐推荐。
Of note, the development of Machine Learning at Spotify has closely tracked academic research into content recommenders and neural networks and from the early days of Collaborative Filtering in 2006 to the latest in Generative Adversarial Networks (GANs), with coherent music generation possible through LTSM (Long Short-Term Memory).
值得注意的是,Spotify的机器学习的发展密切跟踪了对 内容推荐器和神经网络的 学术研究 ,从2006年的协作过滤的早期到最新的生成对抗网络 ( GAN ),通过LTSM可以产生连贯的音乐( 长短期记忆 )。
You can read more about the technical details of how Spotify’s “Paved Road” machine learning infrastructure is implemented through a collection of open-source software platforms like Google Cloud, TensorFlow, and Kubeflow.
您可以阅读有关如何通过一系列开源软件平台(例如Google Cloud , TensorFlow和Kubeflow )实现Spotify的“铺装之路”机器学习基础架构的技术细节的更多信息 。
Let’s do a quick comparison with Netflix — with a market cap of 200 Billion is about 5 times larger than that of Spotify — whose content recommendation system is also a byproduct of Machine Learning.
让我们与Netflix进行快速比较-Netflix的市值为2000亿美元,是Spotify的5倍左右-其内容推荐系统也是Machine Learning的副产品。
According to this article by Spotify’s VP of Engineering: “Netflix has about 158 million users, and at Spotify, we have 248 million. On top of that Netflix has around 5,800 movies and shows across all territories, while Spotify has more than 50 million music tracks and 500,000 podcast titles.”
根据Spotify工程副总裁的这篇文章 : “ Netflix有大约1.58亿用户,而在Spotify,我们有2.48亿。 最重要的是,Netflix在所有地区拥有约5,800部电影和节目,而Spotify拥有超过5,000万首音乐曲目和500,000个播客标题。”
From a computational complexity perspective, Spotify’s real-time complexity requirements are an order of magnitude larger than that of NetFlix and quickly growing — see the observation above about Spotify’s Playlist generation.
从计算复杂性的角度来看,Spotify的实时复杂性要求比NetFlix 的数量级大一个数量级 ,并且还在Swift增长-请参见上面有关Spotify的播放列表生成的观察。
With its Paved Road approach, Spotify has indeed built one of Tech’s most robust and efficient Machine Learning infrastructure.
通过铺平道路的方式,Spotify确实构建了Tech最强大,最高效的机器学习基础设施之一。
Let’s now shift gears from Product to Business.
现在,让我们将产品从业务转移到业务 。
Spotify的企业发展 (Corporate Development at Spotify)
Corporate Development is an ambiguous industry term, but generally for larger companies, it relates to activities like acquiring other companies (M&A), securing corporate financing, divesting of assets or divisions, and management of the intellectual property (IP).
公司发展是一个模棱两可的行业术语,但通常对于大型公司而言,它涉及诸如收购其他公司( M&A ),获得公司融资 , 剥离资产或部门以及管理知识产权(IP)之类的活动 。
Among the Tech Decacorns (companies with a valuation greater than $10 Billion), Spotify has perhaps one of the most active Corporate Development teams in the industry, with a large part of that activity focused on acquiring startups with novel music tech.
在Tech Decacorns (估值超过100亿美元的公司)中,Spotify可能是行业中最活跃的企业开发团队之一,其中很大一部分专注于收购具有新颖音乐技术的初创公司。
In a similar vein to what we did in “The VC’s Guide to Machine Learning”, we have mapped Spotify’s startup acquisitions with investable themes in the AudioTech industry.
与我们在“ VC的机器学习指南”中所做的类似,我们将Spotify的初创公司收购与AudioTech行业中的可投资主题进行了映射 。

Although music continues to be their core offering, Spotify’s strategic vision is to expand into Audio in general like Podcasting — a thesis reinforced by their numerous acquisitions of Podcasting startups like Anchor, Parcast, and Gimlet.
尽管音乐仍然是他们的核心产品,但Spotify的战略目标是将其广泛地扩展到Podcasting之类的Audio上 。这一论点因其对Anchor , Parcast和Gimlet等Podcasting初创公司的大量收购而得到了加强。
From a thematic perspective, I expect Spotify’s leadership — principally driven by Daniel Ek and his Lieutenant Gustav Söderström — to shift more investment capital to Content Distribution / Licensing / Marketing and “Exploration”.
从主题的角度来看,我希望Spotify的领导层(主要由Daniel Ek和他的中尉古斯塔夫·索德斯特罗姆(GustavSöderström)领导) 会将更多的投资资本转移到内容分发/许可/营销和“探索”上。
I define Exploration broadly as tools and services that enable music (and audio in general) listeners to (1) discover new content and (2) experience audio content in a novel manner.
我将Exploration广泛地定义为工具和服务,这些工具和服务使音乐(通常是音频)的听众能够(1)发现新内容,并且(2)以新颖的方式体验音频内容。
Novel in what way?
小说以什么方式?
With the coming of age of Virtual Reality and Augmented Reality (which is a more challenging problem from a technical perspective), I can see virtual concerts and live “jam sessions” being an active area of investment for Spotify this decade.
随着虚拟现实和增强现实时代的到来(从技术角度来看这是一个更具挑战性的问题),我可以看到虚拟音乐会和现场“干扰会议”是Spotify十年来的活跃投资领域。
Returning now to Allemansrätten where we started.
现在回到我们开始的Allemansrätten 。
最后的想法 (Final Thoughts)
As you may recall Allemansrätten or “Everyman’s Right” is a core tenet of Swedish culture and I believe it is also a core tenet of Decentralization during this Roaring 2020s.
您可能还记得Allemansrätten或“每个人的权利”是瑞典文化的核心宗旨,我相信这也是在2020咆哮之际 下放权力的核心宗旨。
Decentralization — with Blockchain as the enabling technology — will play a key role during this Fourth Industrial Revolution, and unless you get on board the “Allemansrätten train”, you will very likely be missing out on the next Trillion dollar opportunity in Tech.
在第四次工业革命期间 ,以区块链为支持技术的去中心化将发挥关键作用,除非您登上“Allemansrätten火车”,否则您很可能会错过下一个万亿美元的高科技机会。
So if you enjoy the scenic train view, let’s continue Creating Music by Machine Learning.
因此,如果您欣赏风景秀丽的火车,让我们继续通过机器学习来创作音乐 。

A good composer does not imitate; he steals.
优秀的作曲家不会模仿。 他偷了。
— Igor Stravinsky
—伊戈尔·斯特拉文斯基(Igor Stravinsky)
By Andrew Vo, CFA
CFA安德鲁·沃(Andrew Vo)
Investor at Radna Intellectual Ventures
Radna Intellectual Ventures的投资者
Related Link: The VC’s Guide to Machine Learning
相关链接: VC的机器学习指南
关于拉德纳知识投资公司 (About Radna Intellectual Ventures)
Radna Intellectual Ventures is a venture startup studio with a mission to enable the next generation of Deep Technologies or Deep Tech companies, with a focus on Machine Learning, Natural Language Processing, and Blockchain technologies.
Radna Intellectual Ventures是一家风险投资初创公司,其使命是使下一代Deep Technologies或Deep Tech公司成为可能,其重点是机器学习,自然语言处理和区块链技术。

Our General Partner, Andrew Vo, spent a decade in finance, working at some of the largest investment managers like J.P. Morgan, before pursuing the entrepreneurial path. Andrew serves as an Advisor for startup companies focusing on the FinTech and DeepTech sectors. Andrew is a CFA Charterholder and holds a Masters of Science in Computer Engineering from Cornell University, and a Bachelors of Science in Electrical Engineering and Computer Science from the University of California, Berkeley.
我们的普通合伙人安德鲁·沃 ( Andrew Vo)在追求创业之路之前,曾在JP Morgan等一些最大的投资管理公司工作了十年,从事财务工作。 安德鲁(Andrew)担任专注于金融科技和深度科技领域的创业公司的顾问。 Andrew是CFA宪章持有人,拥有康奈尔大学的计算机工程理学硕士学位,以及加利福尼亚大学伯克利分校的电气工程和计算机科学理学学士学位。
Learn more about Radna Intellectual Ventures here.
在此处了解有关Radna Intellectual Ventures的更多信息。
— Published on June 24, 2020
-于2020年6月24日发布
翻译自: https://medium.com/swlh/creating-music-by-machine-learning-4a1837c345af
机器学习诗词创作
http://www.taodudu.cc/news/show-2732554.html
相关文章:
- 机器学习与网络安全(一)
- tt作曲家简谱打谱软件_作曲家入门指南
- 【机器学习】推荐几款很流行的面向 Javascript 的机器学习库
- Guitar Pro2023吉他谱作曲和练习工具
- mysql数据库教程级联_Mysql实现级联操作(级联更新、级联删除)
- mysql 级联复制
- 存储级联模块
- oracle中删除级联方法,Oracle 外键级联删除
- mybatis 级联查询
- java级联_Java构造器:级联调用,调用兄弟构造器
- OpenCV57:级联分类器的训练
- html级联选择器,jquery实现 级联选择器
- mysql级联更新_Mysql实现级联操作(级联更新、级联删除)(转)
- java 级联删除_Mybatis 级联删除的实现
- jpa级联添加_jpa级联(Cascade)操作
- 级联分类
- mysql级联是什么意思_MySql级联操作
- oracle 允许级联删除,oracle系列--级联删除和级联更新
- MyBatis级联查询
- oracle 级联外键,Oracle 级联删除外键
- mysql外键级联操作_Mysql 外键级联
- mysql级联更新优化_mysql级联更新
- html级联选择器,级联选择器
- 级联查询ajax,ajax级联查询
- mysql级联
- oracle级联怎么设置,Oracle级联操作详解
- JavaScript级联链表
- Mybatis级联
- java 级联删除_JPA级联删除
- 级联
机器学习诗词创作_通过机器学习创作音乐相关推荐
- 机器学习 客户流失_通过机器学习预测流失
机器学习 客户流失 介绍 (Introduction) This article is part of a project for Udacity "Become a Data Scient ...
- 使用机器学习预测天气_使用机器学习的二手车价格预测
使用机器学习预测天气 You can reach all Python scripts relative to this on my GitHub page. If you are intereste ...
- 27个机器学习图表翻译_使用机器学习的信息图表信息组织
27个机器学习图表翻译 Infographics are crucial for presenting information in a more digestible fashion to the ...
- 机器学习 线性回归算法_探索机器学习算法简单线性回归
机器学习 线性回归算法 As we dive into the world of Machine Learning and Data Science, one of the easiest and f ...
- 不使用机器学习的机器视觉_使用机器学习为卡通着色
不使用机器学习的机器视觉 监督式机器学习的一个大问题是需要大量的标记数据. 这是一个大问题,尤其是如果您没有标记的数据,即使在充满大数据的世界中,我们大多数人也没有. 尽管少数公司可以访问大量特定种类 ...
- 机器学习模型 非线性模型_调试机器学习模型的终极指南
机器学习模型 非线性模型 You've divided your data into a training, development and test set, with the correct pe ...
- 使用机器学习预测天气_使用机器学习来预测患者是否会再次入院
使用机器学习预测天气 We are in a age where machines are utilizing huge data and trying to create a better worl ...
- 机器学习算法优缺点_用于机器学习的优化算法的优缺点
机器学习算法优缺点 A deep-dive into Gradient Descent and other optimization algorithms 深入研究梯度下降和其他优化算法 Optimi ...
- 机器学习 数据增加_【机器学习】数据降维概述
机器学习领域中所谓的降维就是指采用某种映射方法,将原高维空间中的数据点映射到低维度的空间中. 机器学习主要问题 数据维数 维数 (又称维度) 数学中:独立参数的数目 物理中:独立时空坐标的数目 维度 ...
最新文章
- Elasticsearch之倒排索引
- POJ2253 Frogger(最短路径)
- TF之AutoML之AdaNet框架:AdaNet框架的简介、特点、使用方法详细攻略
- 中科院大牛带你玩转Python数据分析,大厂offer轻松拿!
- python tkinter画布位置_Python Tkinter坐标画布
- Android——倒计时跳转+sharedpreferences
- 推荐系统组队学习——协同过滤
- 贺利坚老师汇编课程74笔记:按下esc键改变显示颜色
- Jquery表单验证 只能输入数字,
- java future模式 所线程实现异步调用
- python 学习之路1-如何入门
- linux vsftpd 配置及使用详解
- Cookie和Session
- python输入年份判断生肖_python年份判断生肖
- 被罗马人称为的三大蛮族去向和苏格拉的独立
- 多态的综合练习(Java)
- 15-VulnHub-Raven 1
- 扫雷小游戏(简易版)
- 小程序开发(一)新建/拉取项目,配置远程仓库
- 术语-BPM:BPM
热门文章
- 程序员必备:Git入门,超详细
- 软考中级 真题 2018年下半年 系统集成项目管理工程师 基础知识 上午试卷
- python实现微信hook_GitHub - zhouxionger/wechathook: 借助微信hook,拦截修改某些call,填充进我们的Python代码,进行微信公众号文章的爬取...
- RK1126从入门到放弃:番外篇(二)Win10 WSL系统下编译buildroot报错不支持SYSV IPC,导致fakeroot无法正常工作
- linux切换桌面的快捷键,SUSE Linux Gnome桌面快捷键整理
- ADOConnection 打开EXCEL
- Python数据分析 | (31) 透视表和交叉表
- visual studio工程文件介绍
- 小程序ibeacon三点定位
- 尝鲜体验win11,附赠win11镜像下载地址