开花算法_数据科学即将开花,但其根源已经存在了很长时间
开花算法
“The future of data analysis can involve great progress, the overcoming of real difficulties, and the provision of a great service to all fields of science and technology. Will it?”
数据分析的未来将涉及巨大的进步,克服实际困难以及为科学和技术的所有领域提供出色的服务。 会吗?
John Tukey, from ‘The Future of Data Analysis’
John Tukey,摘自“数据分析的未来”
In 1962, mathematician John Tukey stated in his paper, The Future of Data Analysis, “For a long time I thought I was a statistician… But as I have watched mathematical statistics evolve… I have come to feel that my central interest is in data analysis…”. He then describes data analysis as “a science, one defined by a ubiquitous problem rather than by a concrete subject”. In these words, Tukey is stating that while mathematics is simply a set of defined, a priori truths; data analysis is an empirical science where knowledge can be gained through constant experimentation. Tukey appears to more or less define statistics as a subset of mathematics and data analysis. According to Tukey, “Individual parts of mathematical statistics must look for their justification toward either data analysis or pure mathematics”.¹
1962年,数学家约翰·图基(John Tukey)在他的论文《数据分析的未来》中说:“很长一段时间以来,我都以为自己是统计学家……但是当我看着数学统计学的发展……我逐渐感到我对数据的兴趣浓厚。分析…”。 然后,他将数据分析描述为“一门科学,它是由普遍存在的问题而不是具体问题所定义的”。 用这些话来说,图基指出,虽然数学只是一组已定义的先验真理,但事实并非如此。 数据分析是一门经验科学,可以通过不断的实验获得知识。 Tukey似乎或多或少地将统计定义为数学和数据分析的子集。 Tukey认为,“数理统计的各个部分都必须寻找其对数据分析或纯粹数学的正当理由”。¹
We can see that Tukey’s sentiments echo today, as the term ‘Data Science’ is becoming an everyday jargon in the tech world. Additionally, many established universities have created ‘Data Science’ degree programs only in the past half-decade. Many of these programs serve as an extension of their already existing statistics curriculums. It’s safe to say that the field has very quickly become its own unique discipline. Tukey closes his paper with a prescient understanding of the importance data will play in the future as computational storage power becomes greater: “…there are situation[s] where the computer makes feasible what would have been wholly unfeasible… where speed and economy of delivery of answer make the computer essential for large data sets and very valuable for small sets”.¹
我们可以看到Tukey的观点在今天回荡,因为“数据科学”一词正在成为科技界的日常用语。 此外,许多知名大学仅在过去的五年中才创建了“数据科学”学位课程。 其中许多程序是其现有统计课程的扩展。 可以肯定地说,该领域已Swift成为其自己的独特学科。 Tukey在他的论文结尾时,先行地了解了随着计算存储能力的提高,未来数据的重要性:“…在某些情况下,计算机使可行的事情变得完全不可行……在交付速度和经济性方面答案使计算机对于大型数据集必不可少,而对于小型数据集则非常有价值。”¹
Fast-forward to 1994. BusinessWeek publishes an article titled Database Marketing. The article goes into detail describing the rise of new checkout scanner technologies that were being used in stores during the eighties. The vision was that each scanner would create a transaction record that stored data on each item purchased. This could then, in turn, give retailers insights on what to advertise based on customer demand and purchase history.² In the end, however, this checkout scanner craze didn’t live up to its promise. Companies simply didn’t have the technological infrastructure or the computational power to handle these volumes of data, let alone get insights from them.³
快进到1994年。《商业周刊》发表了一篇名为《数据库营销》的文章。 本文详细介绍了八十年代在商店中使用的新型结帐扫描仪技术的兴起。 愿景是每个扫描仪都会创建一个交易记录,以存储有关所购买的每个项目的数据。 这样一来,零售商就可以根据客户需求和购买历史来做广告。²但是,最终,这种结帐扫描仪热潮并没有实现其承诺。 公司根本没有处理这些海量数据的技术基础架构或计算能力,更不用说从中获取见解了。³
However, it’s important to note that this experiment, even though it failed, represented an early version of a very important concept. It represented a vision of utilizing massive-scale analytics to predict customer desires. And this was back in the eighties, when the internet wasn’t even mainstream yet. In fact, by the nineties, some companies were able to use this sort of tech while it was in its infancy. Blockbuster Video, for example, used its database of memberships and transactions to test a computerized system to recommend movies based on prior rentals.² It’s wild to think that Blockbuster, of all companies, was an early researcher into a content recommendation algorithm!
但是,必须注意的是,即使这个实验失败了,它还是一个非常重要的概念的早期版本。 它代表了一种利用大规模分析来预测客户需求的愿景。 这可以追溯到八十年代,当时互联网还不是主流。 实际上,到90年代,一些公司还可以在婴儿期使用这种技术。 例如,百视达视频使用其会员和交易数据库来测试计算机系统,以根据先前的租金来推荐电影。²疯狂地认为,百视达是所有公司中对内容推荐算法的早期研究者!
The following quote from the article almost sounds like it could have been written in an article from this year: “Companies are collecting mountains of information about you, crunching it to predict how likely you are to buy a product, and using that knowledge to craft a marketing message precisely calibrated to get you to do so”.²
这篇文章的以下引述听起来似乎可以写成今年的一篇文章:“公司正在收集有关您的大量信息,对它们进行整理以预测您购买产品的可能性,并利用这些知识来制作产品经过精确校准的营销信息可以帮助您做到这一点。”²
那么,在过去的二十年中,导致数据和分析在几乎每个技术组织中都扮演着至关重要的角色的事情发生了吗? (So what happened in the last two decades that have caused data and analytics to play an essential role in almost every technology organization?)

Well to put it simply, technological capabilities increased exponentially, and in a relatively short period of time. The internet became a mainstream tool used by business and individuals alike. And remember the checkout scanner which collected data? Well in this era, there is basically no barrier to storing mass volumes of data. Today, endless amounts of data are collected and available for analysis at a moment’s notice. And this data stems from countless unique domains. This could be healthcare data, social media data, customer data; the list goes on. And businesses are eager to utilize their computational capacity to analyze this data because it serves as the key to their success.
简单地说,技术能力在相对较短的时间内呈指数增长。 互联网已成为企业和个人使用的主流工具。 还记得结帐扫描仪收集了哪些数据吗? 在这个时代,存储大量数据基本上没有障碍。 如今,无数的数据被收集起来,可在需要时立即进行分析。 这些数据来自无数独特的领域。 这可能是医疗保健数据,社交媒体数据,客户数据; 清单继续。 而且企业渴望利用其计算能力来分析此数据,因为它是成功的关键。
And what about the computational power to store and process this data? Well today, GPU’s are able to process data and execute data-intensive algorithms at speeds exponentially faster than once thought possible.⁴ On top of that, data centers provide warehouses of off-site data storage. Cloud-computing vendors can then offer this storage as-needed to businesses and individuals. And on top of that, completely new paradigms of big data analysis have been created. The most notable project that achieved this was Apache Spark, an open source experiment that began at UC Berkeley’s AMPLab. The advent of Spark completely transformed the landscape of big data computing; as it perfected the paradigm of multi-machine processing. This allowed data to be distributed between multiple clusters and processed in parallel to maximize run-time efficiency.⁵ Additionally, the Spark project was able to perfect the MapReduce programming paradigm through the introduction of Resilient Distributed Datasets (or RDDs) as its fundamental data structure.⁶
那么存储和处理这些数据的计算能力又如何呢? 如今,GPU能够以前所未有的指数级速度处理数据和执行数据密集型算法。⁴最重要的是,数据中心提供了异地数据存储仓库。 然后,云计算供应商可以根据企业和个人的需要提供此存储。 最重要的是 ,已经创建了大数据分析的全新范例。 实现这一目标的最著名的项目是Apache Spark,这是一个从UC Berkeley的AMPLab开始的开源实验。 Spark的出现彻底改变了大数据计算的格局。 完善了多机处理的范式。 这样一来,数据就可以在多个集群之间分配并并行处理,从而最大化运行时效率。⁵此外,Spark项目通过引入弹性分布式数据集(RDD)作为其基本数据结构,能够完善MapReduce编程范例。 .⁶
这些企业的目标到底是什么? 数据如何成为“成功之道”? (What are these businesses’ goals exactly? And how is data the ‘key to their success’?)

Well, the possibilities really are endless. Data Science seeps into practically every domain.⁴ And while the buzzword ‘Machine Learning’ is often associated with the exciting world of artificial intelligence, in the real world it is mostly just a means of giving insights to shareholders. And as mentioned, the use cases are plentiful.
好吧,可能性确实是无止境的。 数据科学几乎渗透到每个领域。⁴虽然流行语“机器学习”通常与令人兴奋的人工智能世界相关联,但在现实世界中,它大多只是一种向股东提供见解的手段。 如前所述,用例很多。
First of all, data can have an immensely positive effect on a company’s advertising strategy. The scientific efficiency of data analytics can save companies advertising dollars. This is because there is less money being wasted on strategies that haven’t been computationally verified.⁷ In the past, you didn’t have the data storing capacity to perform analysis on such large sets. Now, when performing analytics on historical customer and transaction records, companies can be sure that their algorithms are classifying the exact marketing strategies that need to be prioritized.
首先,数据可以对公司的广告策略产生巨大的积极影响。 数据分析的科学效率可以为公司节省广告费用。 这是因为更少的钱被浪费在了未经计算验证的策略上。,过去,您没有数据存储能力来对如此大的数据集进行分析。 现在,在对历史客户和交易记录进行分析时,公司可以确保其算法对需要确定优先级的确切营销策略进行了分类。
Next, consider the medical field. Perhaps a pharmaceutical company wants to predict the likeliness of a new drug being adopted. Then they can mine through historical claims data and create a predictor based on diagnosis patterns across various demographic attributes.
接下来,考虑医学领域。 也许某制药公司希望预测采用新药的可能性。 然后,他们可以挖掘历史索赔数据并根据各种人口统计特征的诊断模式创建预测变量。
Data can even make strides in airline safety. For example, Southwest Airlines and NASA have teamed up on a text-mining project to identify potential hazards by studying air traffic control transcripts and data content generated by airplanes.⁸
数据甚至可以大大提高航空公司的安全性。 例如,西南航空(Southwest Airlines)和美国国家航空航天局(NASA)合作开展了一项文本挖掘项目,通过研究空中交通管制记录和飞机产生的数据内容来识别潜在危害。
I’ll stop listing use cases for now. But the point is, I could keep on going forever if I wanted to. You could write an encyclopedia on each business domain and use case Data Science influences. Its effect on organizational goals has truly been that profound. Whether a business’ goals involve increasing ROI or promoting the public well-being, data will play a role in some shape, way, or form.
我将暂时停止列出用例。 但是关键是,如果我愿意的话,我可以永远继续下去。 您可以在每个业务领域和用例数据科学的影响下编写百科全书。 它对组织目标的影响确实如此深远。 无论企业的目标涉及增加ROI还是促进公众福祉,数据都将以某种形式,方式或形式发挥作用。
撇开:人工智能与自动化 (Aside: AI versus Automation)

The above use cases are more in line with AI, machine learning, and classification. But before we move on to use cases for automation, it’s important to note that automation is not to be confused with AI. While AI (and its subset, machine learning) is meant to mimic what a human can identify; automation is meant to continuously mimic tasks that a human can do. In other words, while AI algorithms have to do with classifying insights, automation algorithms have to do with continuously simulating repetitive tasks.
上面的用例更符合AI,机器学习和分类。 但是,在继续进行自动化用例之前,必须注意不要将自动化与AI混淆。 人工智能(及其子集,机器学习)旨在模仿人类可以识别的东西 ; 自动化意味着不断模仿人类可以完成的任务。 换句话说,虽然AI算法必须与对见解进行分类有关,但自动化算法必须与不断模拟重复性任务有关。
However, we must also consider the fact that the two are not necessarily mutually exclusive, and they oftentimes work together hand-in-hand. The best use case to illustrate this idea is self-driving technology. In this case, you are automating the task of driving uninterrupted for long periods of time. However, the task is not as menial as a simple copy-and-paste. There are many extraneous factors such as traffic lights, signs, and other vehicles. This is where AI gets supplemented into the mix. The car will need to implement classifiers to watch out for these extraneous factors and learn how to react to them.
但是,我们还必须考虑以下事实:两者不一定是互斥的,而且它们有时常常携手并进。 可以说明这种想法的最佳用例是自动驾驶技术。 在这种情况下,您要使长时间不间断驾驶的任务自动化。 但是,该任务并不像简单的复制和粘贴那样艰巨。 有许多无关紧要的因素,例如交通信号灯,标志和其他车辆。 这是将AI补充到其中的地方。 汽车将需要实施分类器,以注意这些无关紧要的因素,并学习如何对它们做出React。
Later on, when we get to the topics of data policy and strategy, I’ll probably use the two terms interchangeably. Because the two terms are indeed different, but they’re heavily related. Now that we’ve cleared this confusion, let’s move on to more business goals.
稍后,当我们谈到数据策略和策略主题时,我可能会互换使用这两个术语。 因为这两个词确实是不同的,但是它们是密切相关的。 既然我们已经清除了这种困惑,那么让我们继续进行更多的业务目标。
企业希望通过自动化实现哪些成果? (What are some outcomes business’ seek to achieve with automation?)
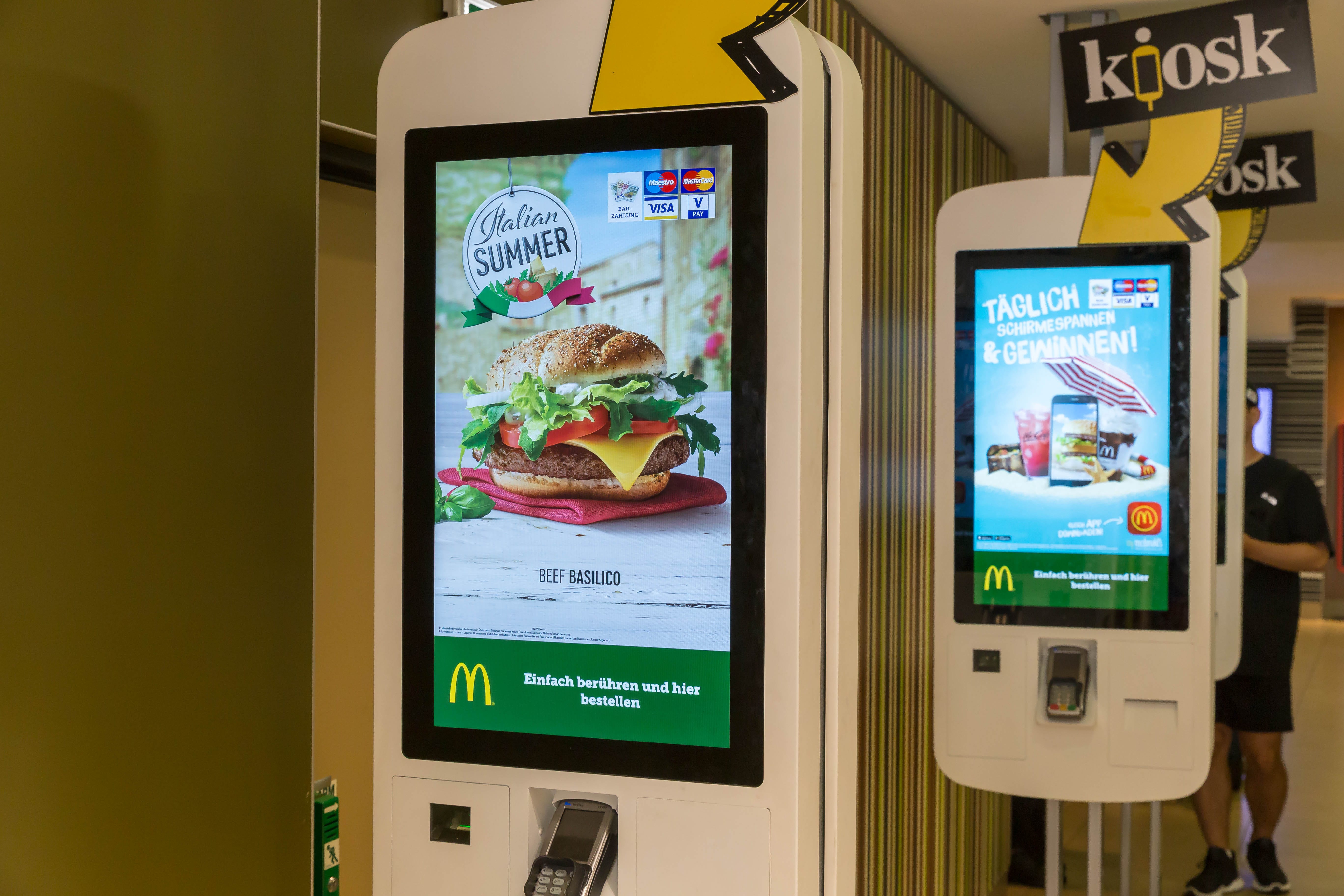
The key goals with automation are pure efficiency and productivity. According to McKinsey, automation alone could raise annual global productivity from 0.8% to 1.4%. And several labor sectors are already utilizing automation. For example, the Australian mining company Rio Tinto has rolled out automated haul trucks and drilling machines which increased productivity drastically.⁴
自动化的主要目标是纯粹的效率和生产力。 麦肯锡认为,仅自动化一项就可以将全球年生产率从0.8%提高到1.4%。 并且一些劳动部门已经在利用自动化。 例如,澳大利亚矿业公司力拓(Rio Tinto)推出了自动牵引车和钻Kong机,从而大大提高了生产率。⁴
As for long-haul trucking, it could well be the next labor sector that is immensely disrupted by automation. Consider the fact that 70% of America’s goods are transported via long-haul trucks.⁹ If you could automate all trucks with self-driving technology and get them to continuously run uninterrupted, then you would have an immensely efficient supply chain once though improbable. Industries like these which rely on purely labor-intensive tasks will always see an increase of efficiency with automation technologies.
至于长途卡车运输,很可能是下一个受自动化极大影响的劳动力部门。 考虑一下美国有70%的货物是通过长途卡车运输的事实。⁹如果您可以使用自动驾驶技术使所有卡车实现自动化,并使它们连续不断地运行,那么即使不可能,您也将拥有一条非常高效的供应链。 诸如此类仅依靠劳动密集型任务的行业,总会发现自动化技术的效率不断提高。
But automation can even apply to industries that require more interpersonal communication. Take the domain of customer service for example. It is estimated that a majority of customer service interactions are now automated.¹⁰ Amazon and Citibank are just a couple of major corporations whose customer service infrastructures rely on virtual assistants to some extent. Customer service automation is also being heavily implemented in the food service industry. McDonald’s for instance, made a plan in 2018 to add self-service kiosks to one-thousand stores each quarter into 2020.¹¹ Today, we see the result, as kiosks are extremely commonplace in their restaurants. It doesn’t matter if a job requires a social aspect or not. Automation is set to disrupt it in some way.
但是自动化甚至可以应用于需要更多人际交流的行业。 以客户服务领域为例。 据估计,现在大多数客户服务交互都是自动化的。¹亚马逊和花旗银行只是几家大型公司,其客户服务基础架构在一定程度上依赖虚拟助手。 客户服务自动化也正在食品服务行业中得到广泛实施。 例如,麦当劳(McDonald's)在2018年制定了计划,到2020年每个季度将自助服务亭增加到一千家。¹¹今天,我们看到了结果,因为自助亭在其餐厅中极为普遍。 一项工作是否需要社交方面都没有关系。 自动化将以某种方式破坏它。
我们完成了业务目标。 但是“第四次工业革命”可能带来哪些负面影响? (We went over business goals. But what are some possible negative implications of the ‘Fourth Industrial Revolution’?)

Oftentimes, we see the advent of AI being associated with the bleak. We often hear that many of the essential, labor-intensive jobs such as factory work and long-haul truck driving will soon be replaced by AI. This is surely an important ethical implication to consider. While past industrial revolutions created new jobs and displaced old ones, the AI revolution appears to be set to eliminate certain sectors completely. The jobs that are set to replace them, are predicted to be heavy in math, computation, and critical analysis. These white-collar jobs are a world away from the labor-intensive blue-collar ones they will soon replace.
通常,我们看到AI的出现与萧条有关。 我们经常听到,许多重要的劳动密集型工作,例如工厂工作和长途卡车驾驶,很快就会被AI取代。 这无疑是要考虑的重要的伦理含义。 过去的工业革命创造了新的工作岗位并取代了旧的工作岗位,而AI革命似乎将彻底消除某些部门。 预计将要替换的工作在数学,计算和批判性分析方面都很繁重。 这些白领工作与即将取代的劳动密集型蓝领工作世界不同。
And training the old workforce will be difficult, not only because they are adapting to an entirely new skill-set; but because they may not have an interest in learning these new skills at all. Consider the fact that the average truck driver in the United States is a middle-aged man nearing retirement, and probably without a college degree.¹² At this age, these people probably have no desire to learn to program. On top of that, they’re at the point in their lives where this job is a very important part of their identity. These are all ideas that need to be addressed when we do experience the AI revolution. And I would go so far as to say that governments need to develop an AI strategy in response to these phenomena.
而且,对旧劳动力进行培训将很困难,不仅因为他们正在适应全新的技能; 但是因为他们可能根本没有兴趣学习这些新技能。 考虑一下这样一个事实,即美国的普通卡车司机是即将退休的中年男子,并且可能没有大学学位。¹²在这个年龄,这些人可能没有学习编程的愿望。 最重要的是,他们正处在人生的关键时刻,这份工作是他们身份的重要组成部分。 当我们经历AI革命时,这些都是必须解决的想法。 我要说的是,政府需要针对这些现象制定AI战略。
政府和公司如何共同实施AI战略? (How can governments and companies work together to implement an AI strategy?)

We’ve been extensively discussing AI and automation through the lens of their main advantage: productivity. But now we must reconcile this with potential ethical implications. According to McKinsey, policymakers actually have a great incentive to embrace these technologies for the well-being of both their economy and their constituents: “This [productivity growth] will help ensure future prosperity, and create the surpluses that can be used to assist workers and society adapt to these rapid changes”.⁴
我们一直在围绕AI和自动化的主要优势(生产力)展开广泛讨论。 但是现在,我们必须将其与潜在的道德影响相协调。 麦肯锡认为,决策者实际上具有极大的动力去拥抱这些技术,以促进其经济和选民的福祉:“这种[生产力的增长]将有助于确保未来的繁荣,并创造可用于帮助工人的剩余资金。和社会适应这些快速变化。”⁴
In other words, productivity and efficiency can cause surplus and prosperity. And as speedy output of goods and services increases, economic surplus will be generated in both the private and public sectors. McKinsey brings up this idea of “public-private” partnerships that can lift developing countries out of poverty through digitization.⁴ But I would go a step further, and say that a partnership of this sort could aid developed countries just as much.
换句话说,生产力和效率会导致过剩和繁荣。 而且,随着商品和服务的快速产出增加,私营和公共部门都将产生经济盈余。 麦肯锡提出了“公私合作”的想法,这种合作可以通过数字化使发展中国家摆脱贫困。⁴但我走得更远,并说这种伙伴关系可以为发达国家提供同样的帮助。
After all, more private revenue from these projects could mean more tax revenue for the government, which can then get pumped back into the people through various government-run initiatives. Remember the concern about certain blue-collar workers being permanently displaced? The government may be able to use this money to provide those displaced workers with social safety nets or a universal basic income.⁴ Perhaps some of these displaced workers can be given the option to participate in some government-sponsored STEM training program. Better yet, these programs can be offered to young students as well to prepare them for a growing workforce which will be in desperate need for new talent.
毕竟,这些项目带来的更多私人收入可能意味着政府可以获得更多税收,然后可以通过各种政府运作的举措将其重新注入人民手中。 还记得某些蓝领工人永久流离失所的问题吗? 政府也许能够使用这笔钱为流离失所的工人提供社会安全网或普遍的基本收入。⁴也许其中一些流离失所的工人可以选择参加一些政府资助的STEM培训计划。 更好的是,这些计划也可以提供给年轻学生,为他们准备急需新人才的不断增长的劳动力做好准备。
Countries all over the world are already implementing AI strategy. In 2018, Korea pledged $2 billion to the creation of AI research, jobs, talent, and government partnerships with “start-ups and corporations in the field [of AI]”.¹³ And in the same year, Google opened Africa’s first AI research facility in Ghana to commit to “collaborating with local universities and research centers, as well as working with policy makers on the potential uses of AI in Africa”.¹⁴ While the AI revolution does indeed have some dreary implications; if implemented correctly, it can provide a new cycle of prosperity where businesses, the state, and citizens all exchange ideas and revenue.
全世界的国家已经在实施AI战略。 2018年,韩国承诺提供20亿美元,用于与“ [人工智能]领域的初创企业和公司”建立人工智能研究,就业,人才和政府合作伙伴关系。¹³同年,谷歌开展了非洲首个人工智能研究位于加纳的工厂致力于“与当地大学和研究中心合作,并与决策者合作,探讨非洲在人工智能方面的潜在用途”。¹尽管人工智能革命确实带来了沉闷的影响; 如果实施得当,它可以提供一个新的繁荣周期,使企业,国家和公民都可以交流思想和收益。
正如约翰·图基(John Tukey)所说,什么是“数据分析的未来”? (What is ‘The Future of Data Analysis’, as John Tukey stated?)

Data, AI, and automation are poised to be the greatest disruptors in technology since computers and the internet. They won’t only disrupt the technological sphere, but will also determine how policy will be dictated for years to come. We explored the idea that the quick rise in technological capabilities jump-started the age of data. But this begs the question: how were these data-driven technologies able to rise so uniformly across all industries and businesses?
数据,人工智能和自动化有望成为自计算机和互联网以来最大的技术颠覆者。 它们不仅会破坏技术领域,还将决定未来几年如何制定政策。 我们探讨了技术能力的Swift提高推动数据时代开始的想法。 但这引出了一个问题:这些数据驱动技术如何在所有行业和企业中如此统一地崛起?
Well every company is trying to be at the forefront of what’s new in technology. Amazon made e-commerce mainstream. As a result, brick-and-mortar companies began to invest heavily in their own e-commerce operations in order to keep up. Similarly, tech giants such as Google, Facebook, and LinkedIn obviously have extremely robust data infrastructures. And this pushed companies like Walmart, the king of brick-and-mortar outlets, to develop their own data strategy. It’s no wonder that the percentage of job starters in analytics and data science increased ten-fold from 1990 to 2010.¹⁵ And this is only going to increase.
每个公司都试图在技术新领域中走在前列。 亚马逊使电子商务成为主流。 结果,实体公司开始大量投资于自己的电子商务运营,以跟上步伐。 同样,诸如Google,Facebook和LinkedIn等技术巨头显然拥有极其强大的数据基础架构。 这促使像实体商店之王沃尔玛这样的公司制定了自己的数据战略。 毫不奇怪,从1990年到2010年,分析和数据科学领域的工作起步者比例增加了十倍。¹而且这只会增加。
I’ll end with a prophetic quote by Google Chief Economist Hal Varian from 2009: “I keep saying the sexy job in the next ten years will be statisticians. People think I’m joking, but who would’ve guessed that computer engineers would’ve been the sexy job of the 1990s? The ability to take data — to be able to understand it, to process it, to extract value from it, to visualize it, to communicate it — that’s going to be a hugely important skill in the next decades…”.¹⁶ And it’s true. Currently, there are many students studying to prepare for jobs that don’t even exist yet. The mainstream nature of data, AI, and automation are relatively new. And once these jobs do come to fruition; they will be plentiful, and essential to the operation of our entire society.
最后,我将以谷歌首席经济学家哈尔·瓦里安(Hal Varian)从2009年开始的预言作为结尾:“我一直说,未来十年的工作将是统计学家。 人们以为我在开玩笑,但是谁能想到计算机工程师会成为1990年代最性感的工作呢? 拥有数据的能力-能够理解,处理数据,从中提取价值,进行可视化并进行通信-在接下来的几十年中,这将是一项极为重要的技能……”。⁶ 。 当前,有很多学生正在学习以准备尚不存在的工作。 数据,人工智能和自动化的主流性质相对较新。 一旦这些工作成真, 它们将是充足的,并且对于我们整个社会的运作至关重要。
Citations & Sources:
引用与来源:
[1]: Tukey, John W. “The Future of Data Analysis.” The Annals of Mathematical Statistics, vol. 33, no. 1, 1962, pp. 1–67., doi:10.1214/aoms/1177704711.
[1]:Tukey,JohnW。“数据分析的未来。” 数理统计年鉴 。 33,没有 1962年1月,第1至67页,doi:10.1214 / aoms / 1177704711。
[2]: Berry, Jonathan. “Database Marketing.” Bloomberg.com, Bloomberg, 5 Sept. 1994, www.bloomberg.com/news/articles/1994-09-04/database-marketing.
[2]:贝瑞,乔纳森。 “数据库营销”。 彭博社 ,彭博社,1994年9月5日, www.bloomberg.com / news / articles / 1994-09-04 / database-marketing。
[3]: Press, Gil. “A Very Short History Of Data Science.” Forbes, Forbes Magazine, 15 Oct. 2014, www.forbes.com/sites/gilpress/2013/05/28/a-very-short-history-of-data-science/.
[3]:按,吉尔。 “数据科学史非常短。” 福布斯》 ,《福布斯》杂志,2014年10月15日, www.forbes.com / sites / gilpress / 2013/05/28 / a-very-short-history-of-data-science /。
[4]: “What’s Now and next in Analytics, AI, and Automation.” McKinsey.com, McKinsey & Company, 11 May 2019, www.mckinsey.com/featured-insights/digital-disruption/whats-now-and-next-in-analytics-ai-and-automation.
[4]:“分析,人工智能和自动化的现在和下一步。” 麦肯锡公司,麦肯锡公司,2019年5月11日, www.mckinsey.com / featured-insights / digital-disruption / whats-now-and-next-in-analytics-ai-and-automation。
[5]: Chambers, Bill, and Matei Zahari. Spark: The Definitive Guide. O’Reilly, 2018.
[5]:钱伯斯,比尔和马泰扎哈里。 Spark:权威指南 。 奥赖利,2018年。
[6]: “Resilient Distributed Dataset (RDD).” Databricks.com, Databricks, 15 May 2020, databricks.com/glossary/what-is-rdd.
[6]:“弹性分布式数据集(RDD)。” Databricks.com ,Databricks,2020年5月15日,databricks.com / glossary / what-is-rdd。
[7]: Agrawal, AJ. “Why Data Is Important for Companies and Why Innovation Is On the Way.” Inc.com, Inc., 24 Mar. 2016, www.inc.com/aj-agrawal/why-data-is-important-for-companies-and-why-innovation-is-on-the-way.html.
[7]:Agrawal,AJ。 “为什么数据对公司很重要,为什么创新正在进行中?” Inc.com ,Inc.,2016年3月24日, www.inc.com / aj-agrawal / why-data-is-important-for-companies-and-why-innovation-is-on-the-way.html 。
[8]: “Data Mining Tools Make Flights Safer, More Efficient.” Nasa.gov, NASA, 2013, spinoff.nasa.gov/Spinoff2013/t_3.html.
[8]:“数据挖掘工具使飞行更安全,更高效。” Nasa.gov,美国航空航天局,2013年,spinoff.nasa.gov/Spinoff2013/t_3.html。
[9]: Wertheim, Jon. “Automated Trucking, a Technical Milestone That Could Disrupt Hundreds of Thousands of Jobs, Hits the Road.” Cbsnews.com, CBS News, 15 Mar. 2020, www.cbsnews.com/news/driverless-trucks-could-disrupt-the-trucking-industry-as-soon-as-2021-60-minutes-2020-03-15/.
[9]:韦特海姆,乔恩。 “自动卡车技术可能会破坏成千上万的工作,这是一个技术里程碑。” Cbsnews.com ,CBS新闻,2020年3月15日, www.cbsnews.com / news / driverless-trucks-could-disrupt-the-trucking-industry-as-soon-as-2021-60-minutes-2020-03- 15 /。
[10]: Schneider, Christie. “10 Reasons Why AI-Powered, Automated Customer Service Is the Future.” Watson Blog, IBM, 16 Oct. 2017, www.ibm.com/blogs/watson/2017/10/10-reasons-ai-powered-automated-customer-service-future/.
[10]:施耐德,克里斯蒂。 “人工智能驱动的自动化客户服务成为未来的10个原因。” Watson Blog ,IBM,2017年10月16日, www.ibm.com / blogs / watson / 2017/10 / 10- reasons-ai-powered-automated-customer-service-future /。
[11]: Hafner, Josh. “McDonald’s: You Buy More from Touch-Screen Kiosks than a Person. So Expect More Kiosks.” Usatoday.com, USA Today, 7 June 2018, www.usatoday.com/story/money/nation-now/2018/06/07/mcdonalds-add-kiosks-citing-better-sales-over-face-face-orders/681196002/.
[11]:哈夫纳,乔什。 麦当劳:您从触摸屏信息亭购买的商品多于个人。 因此,希望有更多信息亭。” Usatoday.com ,今日美国,2018年6月7日, www.usatoday.com / story / money / nation-now / 2018/06/07 / mcdonalds-add-kiosks-citing-better-sales-over-face-face- orders / 681196002 /。
[12]: Kilcarr, Sean. “Demographics Are Changing Truck Driver Management.” Fleetowner.com, FleetOwner, 20 Sept. 2017, www.fleetowner.com/resource-center/driver-management/article/21701029/demographics-are-changing-truck-driver-management.
[12]:基尔卡尔,肖恩。 “人口统计正在改变卡车司机的管理。” Fleetowner.com ,FleetOwner,2017年9月20日, www.fleetowner.com / resource-center / driver-management / article / 21701029 / demographics-are-changing-truck-driver-management。
[13]: Gov’t to Spend 2.2 Trillion Won on National AI Program. Korea JoongAng Daily, 15 May 2018, koreajoongangdaily.joins.com/news/article/article.aspx?aid=3048152.
[13]: 政府不会在国家AI计划上花费2.2万亿韩元 。 韩国中日报,2018年5月15日,koreajoongangdaily.joins.com/news/article/article.aspx?aid=3048152。
[14]: Crabtree, Justina. “Google’s next A.I. Research Center Will Be Its First on the African Continent.” Cnbc.com, CNBC News, 14 June 2018, www.cnbc.com/2018/06/14/google-ai-research-center-to-open-in-ghana-africa.html.
[14]:Crabtree,贾斯汀娜。 “谷歌的下一个AI研究中心将成为其在非洲大陆的第一个研究中心。” Cnbc.com ,CNBC新闻,2018年6月14日, www.cnbc.com/ 2018/06/14/ google-ai-research-center-to-open-in-ghana-africa.html 。
[15]: Patil, DJ. “Building Data Science Teams.” Radar.oreilly.com, O’Reilly, 16 Sept. 2011, radar.oreilly.com/2011/09/building-data-science-teams.html?utm_source=feedburner.
[15]:帕蒂尔,DJ。 “建立数据科学团队。” Radar.oreilly.com ,O'Reilly,2011年9月16日,radar.oreilly.com / 2011/09 / building-data-science-teams.html?utm_source = feedburner。
[16]: “Hal Varian on How the Web Challenges Managers.” McKinsey.com, McKinsey & Company, 1 Jan. 2009, www.mckinsey.com/industries/technology-media-and-telecommunications/our-insights/hal-varian-on-how-the-web-challenges-managers.
[16]:“ Hal Varian谈网络如何挑战管理者。” 麦肯锡公司(McKinsey.com),2009年1月1日, www.mckinsey.com / industries / technology-media-and-telecommunications / our-insights / hal-varian-on-how-the-web-challenges-managers。
翻译自: https://towardsdatascience.com/data-science-is-about-to-blossom-but-its-roots-have-been-here-for-a-very-long-time-e1f05be0774e
开花算法
http://www.taodudu.cc/news/show-3298010.html
相关文章:
- 初探OroCRM和捆绑扩展
- 知不足者好学耻下问者自满_对抗开发人员自满情绪的有效方法
- 使用github API的简单github个人资料页面显示应用程序,以及使用bloc模式的flutter...
- 华为开源构建工具_构建开源软件长达5年并以故事为生
- 每个大洲上的国家_700名员工和多个大洲:Alconost如何建立无办公室业务模式
- 机器人工厂参观心得_机器人工厂的建立
- ai人工智能_当AI接手我们的三种情况时
- (9)雅思屠鸭第九天:顾家北100句翻译
- 编程论语 EPIGRAMS IN PROGRAMMING
- 会话管理_优秀会话管理指南
- 炸了!没有任何HTML/CSS ! 纯Python打造一个网站!
- 2021年北京各区高新技术企业认定时间安排,及常见问题解答
- 融通、华能、大唐、招商局、华润、诚通、有研、保利、新兴际华、安能、华录、华侨城、南光、国新等冷门央企大佬
- 全域旅游发展的“首都经验” , 全域旅游的十大融合
- 美通企业日报 | 年轻妈妈经常焦虑人数超过29%;雅诗兰黛集团首次亮相进博会...
- 6.java项目-尚医通(6)
- 11 医院挂号系统【平台前端搭建与首页】
- Avaya陈蔚:新技术优化保险业务流程
- 金融危机下 保险信息化聚焦什么?
- 尚医通_第12章_用户平台首页数据
- 北京面向社会招录消防员900人 将实行全程退出机制
- 北京企业科技研究开发机构认定,奖励100万
- 北京市保险公司名录
- 三大互联网中心:北京、上海、深圳,你 Pick 哪个?
- 罗马仕php30坏了,罗马仕充电宝的插口坏了,应该怎么修?
- 端口(port)和插口(socket)的区别
- TCP/IP 插口层
- vue中slot插口的用法
- 插口层简介(一)
- 视听技术之耳机麦克风二合一接口录音监听完美设置(smartAudio插口配置方法,解决电脑耳机插孔无反应)
开花算法_数据科学即将开花,但其根源已经存在了很长时间相关推荐
- windows xp进入访客_我的WINDOWS XP1启动到“欢迎使用”时要很长时间才进入卓面...
满意答案 mcjds74784 2013.05.18 采纳率:54% 等级:12 已帮助:3859人 在使用Windows XP的过程中,系统速度会随着时间的推移越来越慢,你可重装系统,但重装后 ...
- 5g创业的构想_数据科学项目的五个具体构想
5g创业的构想 Do you want to enter the data science world? Congratulations! That's (still) the right choic ...
- netflix 数据科学家_数据科学和机器学习在Netflix中的应用
netflix 数据科学家 数据科学 , 机器学习 , 技术 (Data Science, Machine Learning, Technology) Using data science, Netf ...
- corba的兴衰_数据科学薪酬的兴衰
corba的兴衰 意见 (Opinion) 目录 (Table of Contents) Introduction介绍 Salary and Growth薪资与增长 Summary摘要 介绍 (Int ...
- 数据科学还是计算机科学_数据科学101
数据科学还是计算机科学 什么是数据科学? (What is data science?) Well, if you have just woken up from a 10-year coma and ...
- r怎么对两组数据统计检验_数据科学中最常用的统计检验是什么
r怎么对两组数据统计检验 Business analytics and data science is a convergence of many fields of expertise. Profe ...
- 数据库面试复习_数据科学面试复习
数据库面试复习 大面试前先刷新 (REFRESH BEFORE THE BIG INTERVIEW) 介绍 (Introduction) I crafted this study guide from ...
- 多元高斯分布异常检测代码_数据科学 | 异常检测的N种方法,阿里工程师都盘出来了...
↑↑↑↑↑点击上方蓝色字关注我们! 『运筹OR帷幄』转载 作者:黎伟斌.胡熠.王皓 编者按: 异常检测在信用反欺诈,广告投放,工业质检等领域中有着广泛的应用,同时也是数据分析的重要方法之一.随着数据量 ...
- R plot图片背景设置为透明_数据科学06 | R语言程序设计模拟和R分析器
模拟simulation ➢概率函数 概率函数通常用来生成特征已知的模拟数据,以及在统计函数中计算概率值. 对于任意分布有四种基本函数: 前缀 作用 d 产生随机数 r 估计概率分布的密度 p 估计累 ...
最新文章
- 我所理解的Spring AOP的基本概念
- python培训学费多少钱-培训python学费多少python学会得多少钱?
- 简单的XML和JSON数据的处理
- 正则表达式匹配两个特殊字符中间的内容
- 取两个数较小值c语言_如何提升C语言安全性,达到RUST的安全性
- gitlab备份及恢复
- Android之MVVM框架 - 数据绑定
- visual studio code安装_Deepin系统中如何安装Visual Studio Code
- eclipse报错:“start ruble bundle manager”解决方法
- 一次慢查询暴露的隐蔽的问题
- Cocos2D-x设计模式发掘之二:二段构建模式 -----------cocos2d-x3.0正式版本(7.22)
- 开源播放器内核比较:ijkPlayer、VLC、ExoPlayer、MediaPlayer、SmartPlayer
- VS2017使用C#编写程序员告白小软件
- python实现随机抽奖游戏
- 怦然心动(Flipped)-2
- 菜农谋略:搞定牛人宋俊德,对女牛人孙昌旭说:“记住,俺是雁塔菜农~~~ ”
- 为什么能力越低的人,越容易产生对自己过高的评价?
- Datawhale组队学习——Pytorch进阶训练技巧
- 禁用键盘上的某些按键
- 云队友丨40岁之前,希望你懂得这5条定律